The Sales Forecasting and Development of a Statistical Website for P.E. Paper Co., Ltd.
Keywords:
Sales Forecasting, Association Rules, Time Series Analysis, Website Development, CRISP-DMAbstract
This research presents a comprehensive approach to developing and implementing a sales forecasting system alongside a statistical website for P.E. Paper Co., Ltd. The study has three main objectives: first, to generate accurate forecasts of monthly and yearly sales; second, to identify product co-purchase patterns using association rule mining; and third, to develop a dedicated online platform for displaying the forecasting and analysis results. Adopting the Cross-Industry Standard Process for Data Mining (CRISP-DM), the methodology involves time series analysis for sales forecasting and the Apriori algorithm to uncover items that customers frequently purchase together. Drawing from a dataset of 5,622 sales records collected between 2017 and 2021, the study projects total sales of 21,402,008 THB in 2022 and 21,402,192 THB in 2023, indicating a consistent upward trend. Additionally, the most significant product association demonstrates that customers who buy brown paper (rolls) commonly also purchase perforated paper (rolls), reflecting a 60.54% confidence level. Expert assessments of the system revealed high efficiency (mean = 3.96, SD = 0.84) in both data analysis and website design. Furthermore, a user-satisfaction survey involving 30 participants rated the platform at the highest satisfaction level (mean = 4.52, SD = 0.50). The findings underscore the feasibility and advantages of an integrated forecasting and analytics website in optimizing inventory management and strategic decision-making.
References
Fister, I. et al. (2023). A comprehensive review of visualization methods for association rule mining: Taxonomy, challenges, open problems and future ideas. Expert Systems with Applications, 233, 120901.
Lertpaisarnkornkul, E. (2022). Managing Director, P.E. Paper Co., Ltd. (Personal Interview).
Limlawan, W. et al. (2022). Comparing forecasting methods in order to enhance the accuracy of sales predictions: A case study of a plastic water bottle manufacturing company in Thailand.
Pacharawongsakda, E. (2016). Practical Data Mining with RapidMiner Studio 7. Bangkok: Asia Digital Printing.
Rangkakulnuwat, P. (2019). Time Series Analysis for Economics and Business. Bangkok: Chulalongkorn University.
Royal Thai Police Office. (2012). History of paper. Retrieved from http://policeprinting.police.go.th/ppb/?p=1202
Saesiew, B., & Worarat, S. (2019). Time series forecasting techniques for an animal food production factory. Kasem Bundit University.
Thailand Big Data. (2021). Association Rule. Retrieved from https://bigdata.go.th/big-data-101/data-science/what-is-association-rule/
Tripathy, A., Das, S., & Patra, P. K. (2010). An improved design approach in spatial databases using frequent Association Rule Mining algorithm. 2010 IEEE 2nd International Advance Computing Conference (IACC), pp. 404–409.
Unyuang, J. et al. (2020). Forecasting sales for Pattanapon ABO Part., Ltd.: A statistical and data display program. Bachelor’s degree project, Rajamangala University of Technology Krungthep.
Wang, H. (2011). Time series modeling and predicting for Highway Transport Freight. 2011 International Conference on Remote Sensing, Environment and Transportation Engineering, pp. 5603–5605.
Zhang, M. (2023). Financial Time Series Frequent Pattern Mining Algorithm Based on Time Series ARIMA Model. 2023 International Conference on Networking, Informatics and Computing (ICNETIC), pp. 244–247.
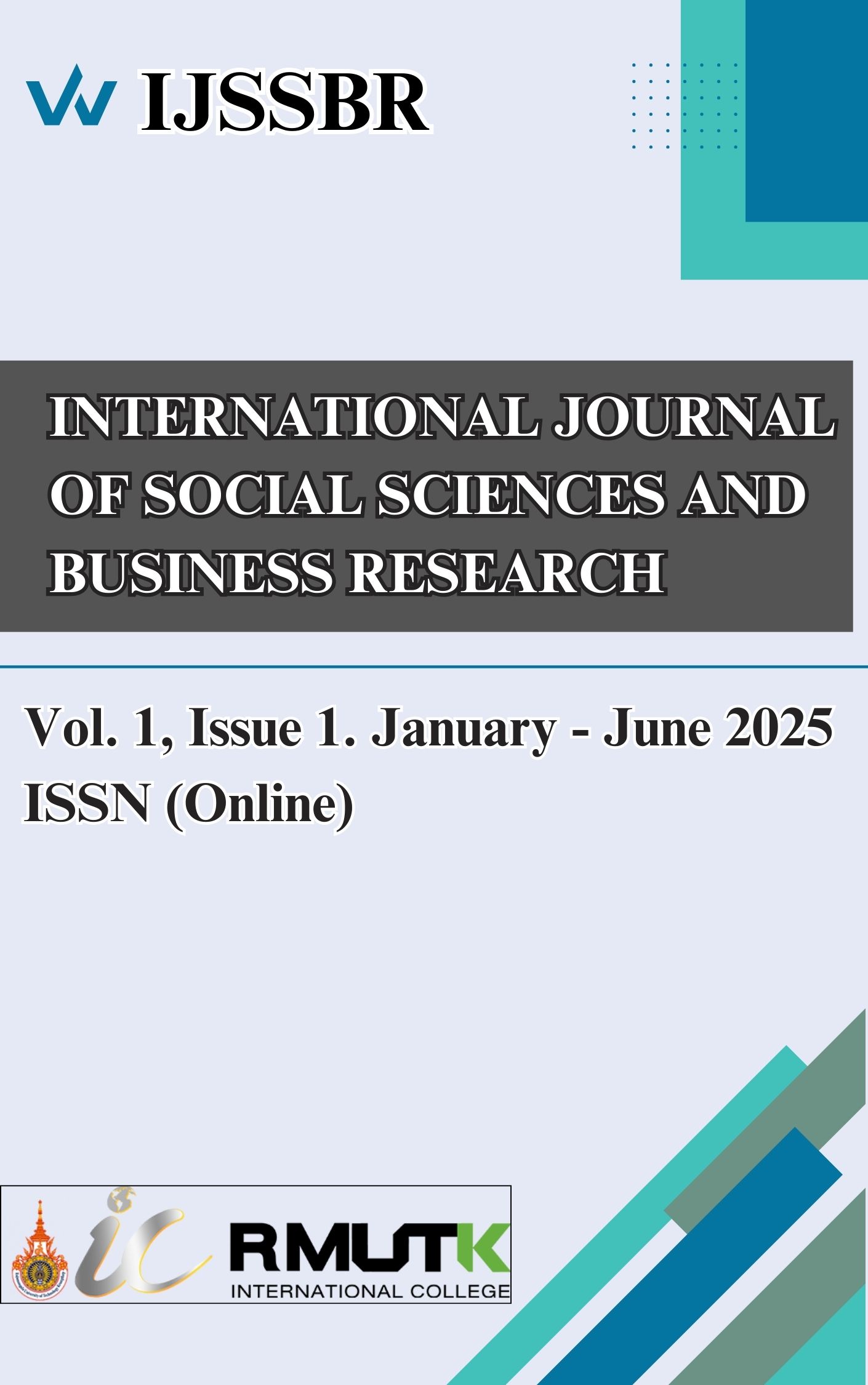
Downloads
Published
How to Cite
Issue
Section
License
Copyright (c) 2025 Rajamangala University of Technology Krungthep

This work is licensed under a Creative Commons Attribution-NonCommercial-NoDerivatives 4.0 International License.